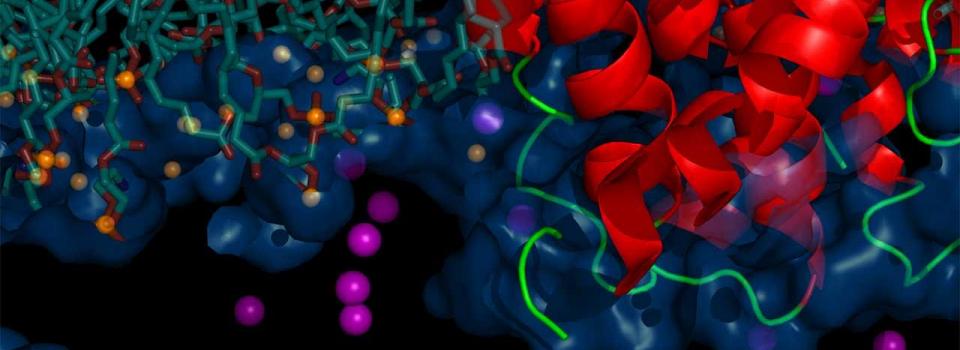
The seminar is sponsored by NYU-ECNU Center for Computational Chemistry at NYU Shanghai
For more information, please see the flyer here: Click>>
Abstract:
One of the challenges of present and near future conversion, storage, and transportation of energy is its catalytic transformation into fuels. The widespread description and modelling of heterogeneous catalysis focus on formation energies and energy barriers, however the free energy at realistic conditions (e.g., temperature and pressure) and the dynamics are also important. Evaluation of thermodynamics quantities via simple harmonic models or more elaborate statistical-mechanics-ensemble sampling allows us to determine phase diagrams and access average populations in disordered systems, from fully ab initio calculations. I show how sampling methods like replica exchange molecular dynamics, global sampling algorithms like genetic algorithms, together with thermodynamic modelling, can be successfully applied to predict observable properties of systems like MgO clusters, MgO defected surfaces, TiO2 clusters, and gold clusters. For a meaningful representation of thermodynamic (and dynamical) properties from atomistic calculations, it is vital to choose representative parameters that determines the quantity or process under study. These parameters are called descriptor in statistical learning. Here, I show a proof-of-concept application in which feature-extraction tools borrowed from statistical (machine) learning can be applied to the prediction of properties of materials.
Biography:
Luca Massimiliano Ghiringhelli has been since 2011 a group leader in the theory group of the Fritz Haber Institute. His background is in computational statistical mechanics and electronic structure methods applied to the evaluation of thermodynamic and kinetic properties of bulk materials, surfaces, and nano-clusters. Recently, he started applying methods inspired to compressed sensing to the modelling of big data in materials science. He is involved in the development of the Novel Materials Discovery Laboratory (http://nomad-coe.eu).