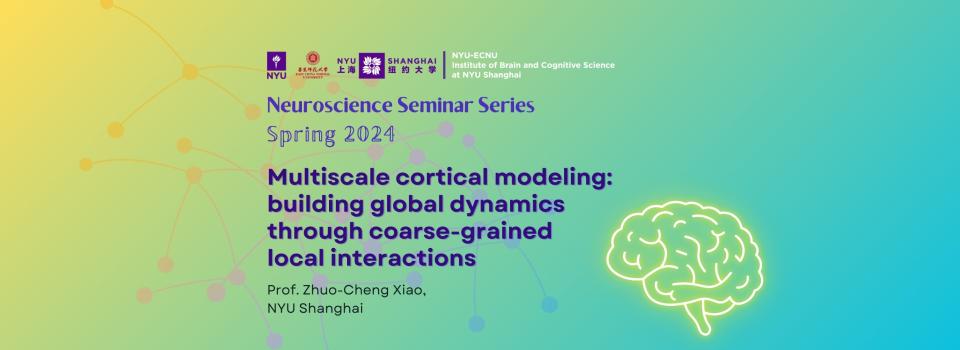
Host: Prof. Xing Tian, NYU Shanghai
Abstract
Modeling the human cortex is challenging due to its structural and dynamic complexity. Biological realistic models can incorporate many details of cortical circuits but are computationally costly and difficult to scale up, restricting the range of phenomena that can be studied. Alternatively, simpler, phenomenological models are easier to build and run but often lose direct comparability with experimental data. This talk introduces a multiscale modeling framework designed to balance biological realism with computational efficiency.
The presentation is structured into two parts, focusing on coarser and finer scales respectively. The first part explains how our multiscale model integrates coarse-grained network representations with an interaction kernel that couples the coarser and finer scales. This interaction kernel is a local response function, translating inputs of a local circuit into outputs—the more accurately it captures local dynamics, the more realistic our model becomes. We precompute a library of potential local responses, allowing fast library lookups to determine the steady states of the modeled cortical area. This approach has accelerated simulations by approximately 2000 times in a detailed model of a layer of primate primary visual cortex (V1).
However, the library's cost becomes prohibitive when modeling multiple brain areas, as the computational demands grow exponentially with input space dimensionality. Therefore, the second part of the talk will introduce our recent advances in a mathematical theory to directly map inputs and parameters to outputs of local circuits. For the first time, we link spiking network dynamics to a set of ordinary differential equations (ODEs), providing a natural framework for mathematical analysis of crucial dynamics emerging from spiking networks. This ODE system quantitatively captures the detailed, transient dynamics, long-term behaviors, and finite-size effects of structurally homogeneous spiking networks. While complementing our multiscale model, potential future applications of ODE theory also include analyzing neural oscillations and metastability in decision-making processes.
Click here to explore Prof. Xiao's biography and research areas.