Mar 12 2015
Published by
NYU Shanghai
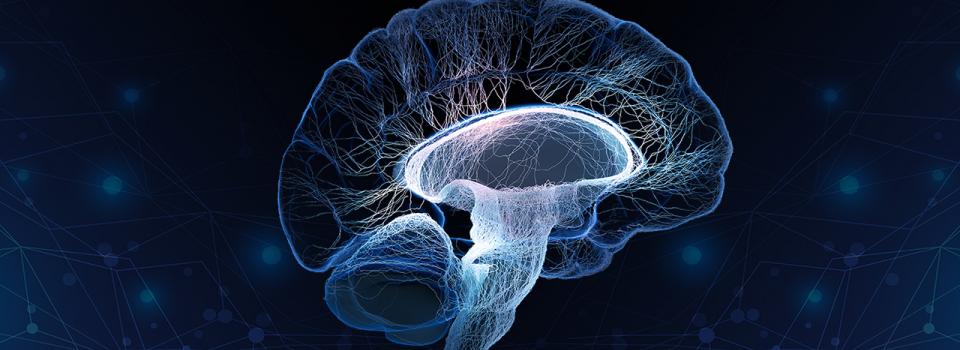
NYU and NYU Shanghai researchers have devised a computer model to explain how a neural circuit learns to classify sensory stimuli into discrete categories, such as "car vs. motorcycle." Their findings, which appear in the journal Nature Communication, shed new light on the brain processes underpinning judgments we make on a daily basis.
"Categorization is vital for survival, such as distinguishing food from inedible things, as well as for formation of concepts, for instance 'dog vs. cat,' and relationship between concepts, such as hierarchical classification of animals," said author Xiao-Jing Wang, Global Professor of Neural Science, Physics, and Mathematics at NYU and NYU Shanghai. "Our proposed model can only explain category learning of simple visual stimuli. Future research is needed to explore if the general principles extracted from this model are applicable to more complex categorizations."
Wang conducted the study with Tatiana Engel, a postdoctoral associate at the time of the study, and Jah Chaisangmongkon, a doctoral candidate in his group, in collaboration with experimentalist David Freedman, a neurobiologist at the University of Chicago. Freedman had previously developed a behavioral paradigm for investigating electrical activity of single-neurons that are correlated with category memberships of visual stimuli.
In this neural-circuit model, which incorporates what we know about the organization and neurophysiology of the cortex, lower-level neural circuits send information about visual stimuli to a higher-level neural circuit where an analog stimulus feature (like the direction of a random pattern of moving dots) is classified into binary categories (A or B). The researchers' results showed that the model captured a wide range of experimental observations and yielded specific predictions that were confirmed by an analysis of single-neuron electrical activity recorded in a category-learning experiment.
Interestingly, the researchers found that learning a correct category boundary (dividing the continuous feature into A and B) requires top-down feedback projection from category-selective neurons to feature-coding neurons.
Since the pioneering work of NYU's J. Anthony Movshon, Stanford’s William Newsome, and others, it has been well known that feature-coding sensory neurons reflect an animal's choice about categorical membership (A or B) of a stimulus in a probabilistic way (quantified as "choice probability"). The common belief was that this is because a category choice is influenced by stochastic, or random, activity of sensory neurons through bottom-up, sensory-to-category pathways.
The new model, reported in the Nature Communications article, suggests a novel interpretation, namely that such "choice probability" results from category-to-sensory, top-down signaling.
This finding offers new insights into feedback projections in the brain whose functional significance had previously been a long-standing puzzle, the researchers noted.
This work was supported by the National Institute of Mental Health (R01MH092927) and the Swartz Foundation.