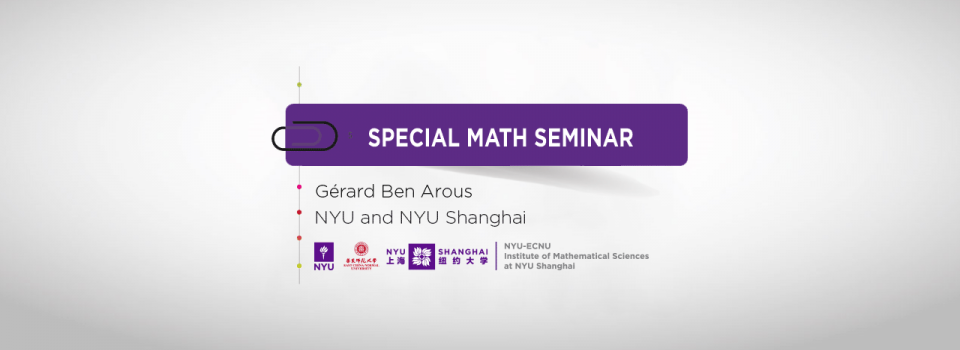
Abstract:
Smooth random functions of many variables can be exponentially complex, form the point of view of Morse Theory, with very many local minima and critical points, and very complex level sets. One consequence of the complexity of these functions is that optimization algorithms could be terribly slow and that it is hopeless to find their global minimum, or any close approximation.
This is the case for instance for random homogeneous polynomials of any degree p>2 on a high dimensional sphere. Just as a teaser for the talks, you could try to find the minimum of a cubic polynomial of N=10^6 variables on the unit sphere, if you pick the coefficients at random (say Gaussian), with whatever computational power and tools you have...
This occurence of complexity for random landscapes is well known in statistical physics, where some of these functions are well studied Hamiltonians of glassy systems, and thus are crucial both for the complex equilibrium behavior,the very slow relaxation to equilibrium and the aging of these systems (the teaser above is called the 3-spin spherical spin-glass).
But these considerations are newer in Machine Learning. After the design step of the network, the core step of most Machine Learning systems is typically the minimization of a loss function. This is a (almost) smooth random function of very many variables. So, why is complexity not a problem there?
Is it simply that the deep learning systems are built on the right side of the so-called “topological transition”? Or maybe that the algorithms used in practice, mostly variants of the SGD, are so much better than the reversible dynamics used in physics that they are able to avoid the difficulty of the landscapes? Or is this a rosy picture and is complexity in fact a problem?
1) We will first discuss the general question of complexity of random functions.The Kac-Rice formula is the well-known mathematical tool to study these questions, and it builds a bridge between these questions of random geometry/topology with deep questions about the spectrum of large random matrices.
2) We will also report on recent work on the dynamical exploration of these landscapes.
3) We will present important examples of the random functions of high dimensional statistics or machine learning and study in depth one important problem amenable to analysis: Tensor PCA.
4) We will conclude by a discussion of very recent numerical results about the efficiency of stochastic gradient descent algorithms for both toy-models and realistic models of deep learning and their comparison to the known behavior of glassy dynamics.
These talks are based on collaborations with mathematicians, statisticians, physicists, and computer scientists: Tuca Auffinger (Northwestern), Marco Baity-Jesi (CEA-Paris), Giulio BIroli (CEA-Paris), Chiara Cammarota (King’s College), Jiri Cerny (Vienna), A. Choromanska(NYU), Reza Ghessairi (Courant), Aukosh Jagannath (Harvard), Yann Le Cun (Facebook and Courant), Song Mei (Stanford), Andrea Montanari (Stanford), Mihai Nica (Toronto), Valentina Ros (CEA-Paris), Levent Sagun (ENS-Paris), Eliran Subag (Courant), Ofer Zeitouni (Weizman and Courant).
Biography:
Gérard Ben Arous, a specialist of probability theory and its applications, has been Professor of Mathematics at NYU's Courant Institute since 2002 and served as its Director and NYU’s Vice Provost for Science and Engineering Development from 2011 to 2016. He now serves as Associate Provost for the Quantitative Disciplines for NYU Shanghai. A native of France, Professor Ben Arous studied Mathematics at École Normale Supérieure and earned his PhD from the University of Paris VII (1981). He has been a Professor at the University of Paris-Sud (Orsay), at École Normale Supérieure, and at the Swiss Federal Institute of Technology (EPFL) in Lausanne, where he held the Chair of Stochastic Modeling. He headed the department of Mathematics at Orsay and the departments of Mathematics and Computer Science at École Normale Supérieure. He also founded the Bernoulli Center, a Mathematics Research Institute, at EPFL.
Professor Ben Arous is a member of the American Academy of Arts and Sciences, a Fellow of the Institute of Mathematical Statistics and an elected member of the International Statistical Institute. He has received various international distinctions, among which a senior Lady Davis Fellowship (Israel), the Rollo Davidson Prize (Imperial College, London), the Montyon Prize (French Academy of Sciences), and is a “chevalier des Palmes Académiques” for his work promoting French culture in New York.
He works on probability theory (stochastic analysis, large deviations, random media and random matrices) and its connections with other domains of mathematics (partial differential equations, dynamical systems), physics (statistical mechanics of disordered media), or industrial applications, like Data Science recently. He is mainly interested in the time evolution of complex systems, and the universal aspects of their long time behavior. He has trained 35 younger colleagues, 20 PhD students and 15 Postdocs, who are now working in academia or industry across the world, from New York to Paris to Caltech or Boston, Lyon, Santiago, Geneva, Montreal, Berlin and Vienna.
Seminar by the NYU-ECNU Institute of Mathematical Sciences at NYU Shanghai