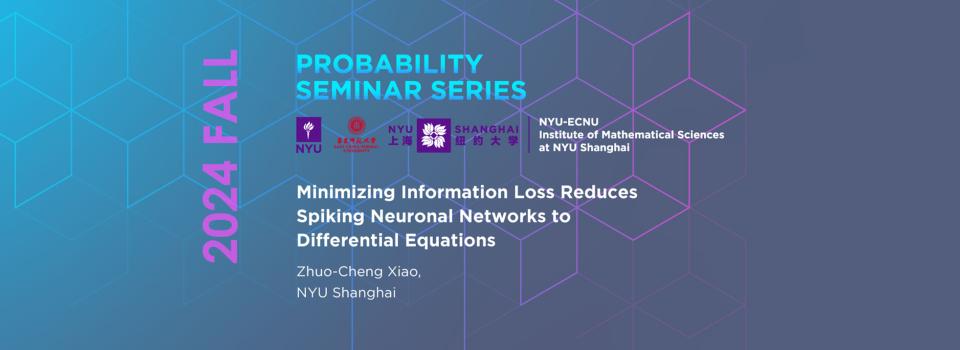
Abstract:
Spiking neuronal networks (SNNs) are widely used in computational neuroscience, from biologically realistic modeling of regional networks in cortex to phenomenological modeling of the whole brain. Despite their prevalence, a systematic mathematical theory for finite-sized SNNs remains elusive, even for homogeneous networks commonly used to represent local neural circuits. The primary challenges are twofold: 1) the rich, parameter-sensitive SNN dynamics, and 2) the singularity and irreversibility of spikes. These challenges pose significant difficulties when relating SNNs to differential equations, leading previous studies to impose additional assumptions or to focus on individual dynamic regimes. In this study, we introduce a coarse-grained Markov approximation of homogeneous SNN dynamics to derive a set of ordinary differential equations (termed "dsODE"). To minimize information loss, our only assumption is the fast self-decorrelation of synaptic conductances. Our dsODE effectively captures dynamical statistics and the geometry of attractors, as well as bifurcation structures under varying parameters. It provides an analytical framework of SNNs by systematically mapping parameters of single-neuronal physiology, network coupling, and external stimuli to SNN dynamics.
Biography:
Zhuo-Cheng Xiao is an Assistant Professor of Mathematics and Neuroscience at NYU Shanghai. He received his Bachelor's degree in Biology from Peking University in 2016 and his Ph.D. in Applied Mathematics from the University of Arizona in 2020. Before joining NYU Shanghai, he was a Swartz Fellow at New York University from 2020-2021 and a Courant Instructor at the Courant Institute of Mathematical Sciences, New York University, from 2021-2023. Dr. Xiao's research spans mathematics and neuroscience, with a focus on developing efficient and biologically realistic computational models of the cortex. His primary research areas include the mathematical theory and computational methods for spiking neural networks.
Seminar by the NYU-ECNU Institute of Mathematical Sciences at NYU Shanghai
This event is open to the NYU Shanghai community and Math community.