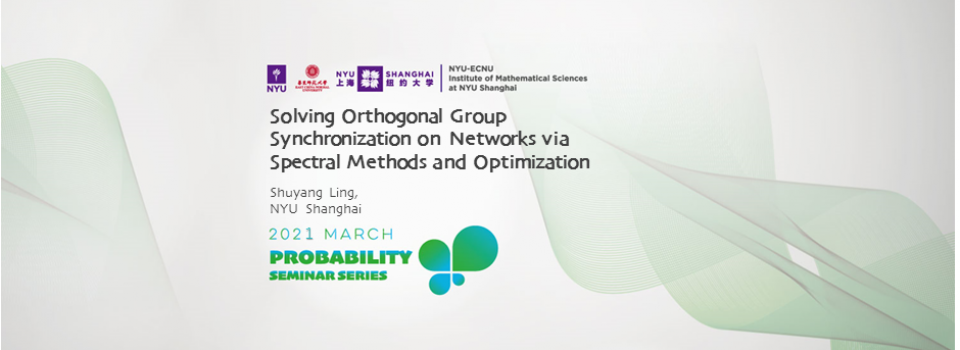
To receive the meeting link, please RSVP for Math
Abstract:
Group synchronization aims to recover the group elements from their noisy pairwise measurements. It has found numerous applications in community detection, clock synchronization, and joint alignment problem. In this talk, we will focus on the orthogonal group synchronization which is often used in cryo-EM, computer vision, and point clouds registration. However, it is generally NP-hard to retrieve the group elements by finding the least squares estimator. We will introduce three different classes of approaches to tackle the orthogonal group synchronization: spectral methods, convex relaxation, and efficient nonconvex method such as Burer-Monteiro factorization and power method. We will discuss several aspects of theoretical and algorithmic advances (a) when do simple spectral methods work. Is it optimal in terms of information theoretical limits? (b) when is convex relaxation tight, i.e., the solution from convex relaxation is exactly the global optimal solution; (c) when does nonconvex approach work? We will understand how the random optimization landscape depends on the signal-to-noise ratio. Numerical experiments will be provided to complement our analysis and future directions will be discussed.
Biography:
Shuyang Ling is an Assistant Professor Faculty Fellow of Data Science at NYU Shanghai. Prior to joining NYU Shanghai, he was a Courant Instructor/Assistant Professor at the Courant Institute of Mathematics and Center for Data Science, New York University, from 2017-2019. Ling's research focuses broadly on the mathematics of data science. He is interested in tackling inverse problems from engineering applications and extracting meaningful information from large-scale and heterogeneous datasets. His research involves a broad spectrum of subjects including optimization, probability, statistics, computational harmonic analysis, and numerical linear algebra.
Seminar by the NYU-ECNU Institute of Mathematical Sciences at NYU Shanghai