Nov 03 2023
Published by
NYU Shanghai
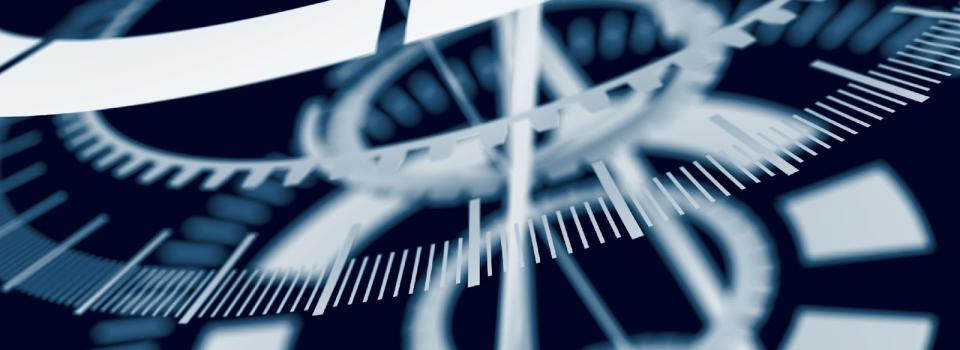
Our lives are governed by countless forms of measurement. In hospitals, medical tests gauge one’s health; in the workplace, interviews assess the right fit for a job candidate, while schools employ exams to evaluate knowledge mastery. In many cases, the results of measurements shape our focus and actions across diverse domains. But how do we measure measurement itself? As the saying goes, "what you measure is what you get.”
A recent study by Professor of Neuroscience Zhong-Lin Lu and his collaborators, published in Nature's Scientific Reports, assesses measurements. They applied a concept from information theory, the expected information gain, to quantify the knowledge gained from different measurements.
"In our lives, measurements are rarely conducted for measurement's sake,” explains Lu. “They serve as guiding tools for subsequent actions.” He gives the example of a patient diagnosed with cancer. A series of measurements are crucial to determine the stage of cancer development, which informs the treatment plan. Therefore, having the 'right' measurements is very important, as it guides or even determines the treatment.
As a vision scientist, Lu has been thinking about how to assess measurements for years, noting that some conventional vision testing methods are no longer as effective as they once were, due to research and scientific development over years. For this study, he drew inspiration from a concept employed in machine learning to address the issue.
The relative magnitude of expected information gain is a common tool in machine learning that is used to select the optimal strategy in the learning process. It’s however not used to quantify the total amount of knowledge obtained through learning, which requires quantification of initial uncertainty before learning. When this concept is introduced to the field of measurement with quantification of initial uncertainty based on the properties of the target population, it aids in evaluating the "value" of different measurements.
The key idea is that expected information gain quantifies the increase in knowledge achieved through measurement, with greater gains when there is more initial uncertainty and when the measurement effectively reduces this uncertainty. In other words, we become more certain about the measurement outcomes with a better measurement.
As the first practical application, the study compared two sets of vision tests - visual acuity (VA) and contrast sensitivity (CS) - using expected information gain. Visual acuity (VA) tests assess the sharpness and clarity of a person's vision, helping diagnose vision problems, determine eyeglass prescriptions, and evaluate suitability for activities like driving or refractive surgery. The results showed that for VA tests, the current gold standard, ETDRS, generated less expected information gain than the active-learning-based qVA test, suggesting potential improvements in VA assessment.
(a) An ETDRS chart (Image courtesy of Precision Vision, Inc). (b) An illustration of qVA.
Contrast sensitivity (CS) is used to detect various eye diseases, such as glaucoma, cataracts, and macular degeneration, by evaluating the ability to perceive subtle changes of light against the background. Study results showed that the CSV-1000 test outperformed the traditional Pelli-Robson test, but the active-learning-based qCSF test demonstrated the highest expected information gain, highlighting its advantages in assessing CS.
(a) A Pelli–Robson chart (Image courtesy of Precision Vision, Inc.). (b) A CSV-1000 chart (Reproduced with permission from GUARDiON Health Sciences, Inc.). (c) An example of stimuli in qCSF test.
The study also emphasizes the importance of considering the target population when comparing expected information gain, as it can vary for different groups. “From our study, we see a tendency that different target populations might require different measurements, and different measurements may have advantages or disadvantages in different populations,” said Lu. “For example, the same questionnaire that can effectively assess the living conditions of the population in Shanghai may not be as accurate when reflecting the population in Zhejiang province.”
"Expected information gain can serve as a valuable tool for objectively evaluating the new knowledge acquired through measurements," said Lu. “It may facilitate better comparisons and informed decision-making in diverse fields involving measurement and data analysis, from scientific research to daily life.”